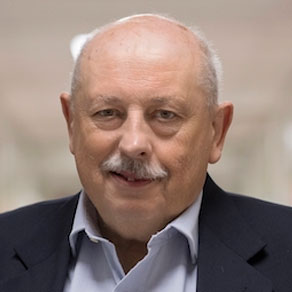
Wladek Minor
[introductory/advanced] Big Data in Biomedical Sciences
Summary
Contemporary scientific research takes advantage of the ever-increasing volume and variety of data to make new discoveries. Similarly, researchers and data scientists/developers working within every sector of society are trying to use vast amounts of data to improve many aspects of our daily lives, including how to make our lives more pleasant and last longer. The ultimate goal in front of us is to transfer gargantuan amounts of data into practical knowledge. This conversion is the real challenge of the 21st century. One of the major impediments to this conversion is that data collected from various sources are sometimes contradictory, and it is not clear how to resolve contrary information without going to the primary source. This situation leads to the lack of reproducibility, the most critical bottleneck of modern research. Experimental reproducibility is the cornerstone of scientific research, and the veracity of scientific publications is crucial because subsequent lines of investigation frequently rely on previous knowledge. Several recent systematic surveys of academic results published in biomedical journals reveal that a significant fraction of representative studies in various fields cannot be reproduced in another laboratory. Artificial intelligence and Big Data approaches are coming to the rescue. The presented lectures aim to discuss a strategy to increase the reproducibility of reported results. Building a set of “best practices” for various ranges of experiments culled by extensive data harvesting is crucial for reproducibility. Experimental verification assisted by automatic/semi-automatic data harvesting from laboratory equipment into the already developed sophisticated laboratory information management system (LIMS) will be presented. The data-in, information-out paradigm will be discussed in detail.
Syllabus
- Big data and big data in Biomedical Sciences
- Why big data is perceived as a big problem – technological considerations
- Data reduction – should we preserve unreduced (raw) data?
- AI role in data reduction
- Databases and databanks
- Data mining in databanks and databases
- Data mining with the use of raw data
- Data integration
- Automatic and semi-automatic curation of large amounts of data
- Amount of data or sophisticated data analysis
- Conversion of databanks into databases and Advanced Information Systems
- Experimental results and knowledge
- Database priorities – content and design
- Interaction of databases with databanks
- Interaction between databases
- Modern data management in biomedical sciences – necessity or luxury
- Automatic data harvesting – close to reality or still on the horizon
- Reproducibility of the biomedical experiments – drug discovery considerations
- Artificial intelligence and machine learning in drug discovery
- Big data in medicine – new possibilities
- Personalized medicine
- Lessons from COVID-19 pandemic
- Can we help local and governmental agencies?
- Future considerations
References
General 1–6, AI and Big Data7–10, Big Data and COVID-199,11–14
- Grabowski M, Minor W (2017) Sharing Big Data. IUCrJ 4:3–4.
- Grabowski M, Cymborowski M, Porebski PJ, Osinski T, Shabalin IG, Cooper DR, Minor W (2019) The Integrated Resource for Reproducibility in Macromolecular Crystallography: Experiences of the first four years. Struct. Dyn. (Melville, N.Y.) [Internet] 6:064301. Available from: http://www.ncbi.nlm.nih.gov/pubmed/31768399
- Grabowski M, Langner KM, Cymborowski M, Porebski PJ, Sroka P, Zheng H, Cooper DR, Zimmerman MD, Elsliger MA, Burley SK, et al. (2016) A public database of macromolecular diffraction experiments. Acta Crystallogr. Sect. D, Struct. Biol. [Internet] 72:1181–1193. Available from: http://www.ncbi.nlm.nih.gov/pubmed/27841751
- Grabowski M, Chruszcz M, Zimmerman MDMD, Kirillova O, Minor W (2009) Benefits of structural genomics for drug discovery research. Infect. Disord. Drug Targets [Internet] 9:459–74. Available from: http://www.pubmedcentral.nih.gov/articlerender.fcgi?artid=2866842&tool=pmcentrez&rendertype=abstract
- Cooper DR, Grabowski M, Zimmerman MD, Porebski PJ, Shabalin IG, Woinska M, Domagalski MJ, Zheng H, Sroka P, Cymborowski M, et al. State-of-the-Art Data Management: Improving the Reproducibility, Consistency, and Traceability of Structural Biology and in Vitro Biochemical Experiments. In: Methods in molecular biology (Clifton, N.J.). Vol. 2199. ; 2021. pp. 209–236. Available from: http://link.springer.com/10.1007/978-1-0716-0892-0_13
- Zheng H, Porebski PJ, Grabowski M, Cooper DR, Minor W (2017) Databases, Repositories, and Other Data Resources in Structural Biology. Methods Mol. Biol. [Internet] 1607:643–665. Available from: http://www.ncbi.nlm.nih.gov/pubmed/28573593
- Brzezinski D, Porebski PJ, Kowiel M, Macnar JM, Minor W (2021) Recognizing and validating ligands with CheckMyBlob. Nucleic Acids Res. [Internet] 49:W86–W92. Available from: https://academic.oup.com/nar/advance-article/doi/10.1093/nar/gkab296/6255698
- Czyzewski A, Krawiec F, Brzezinski D, Porebski PJ, Minor W (2021) Detecting anomalies in X-ray diffraction images using convolutional neural networks. Expert Syst. Appl. [Internet] 174:114740. Available from: https://doi.org/10.1016/j.eswa.2021.114740
- Wlodawer A, Dauter Z, Shabalin IG, Gilski M, Brzezinski D, Kowiel M, Minor W, Rupp B, Jaskolski M (2020) Ligand-centered assessment of SARS-CoV-2 drug target models in the Protein Data Bank. FEBS J. [Internet] 287:3703–3718. Available from: https://onlinelibrary.wiley.com/doi/abs/10.1111/febs.15366
- Kowiel M, Brzezinski D, Porebski PJ, Shabalin IG, Jaskolski M, Minor W (2019) Automatic recognition of ligands in electron density by machine learning. Bioinformatics [Internet] 35:452–461. Available from: http://www.ncbi.nlm.nih.gov/pubmed/30016407
- Grabowski M, Macnar JM, Cymborowski M, Cooper DR, Shabalin IG, Gilski M, Brzezinski D, Kowiel M, Dauter Z, Rupp B, et al. (2021) Rapid response to emerging biomedical challenges and threats. IUCrJ [Internet] 8:395–407. Available from: https://scripts.iucr.org/cgi-bin/paper?S2052252521003018
- Brzezinski D, Kowiel M, Cooper DR, Cymborowski M, Grabowski M, Wlodawer A, Dauter Z, Shabalin IG, Gilski M, Rupp B, et al. (2021) Covid-19.bioreproducibility.org: A web resource for SARS-CoV-2-related structural models. Protein Sci. [Internet] 30:115–124. Available from: http://www.ncbi.nlm.nih.gov/pubmed/32981130
- Shabalin IG, Czub MP, Majorek KA, Brzezinski D, Grabowski M, Cooper DR, Panasiuk M, Chruszcz M, Minor W (2020) Molecular determinants of vascular transport of dexamethasone in COVID-19 therapy. IUCrJ [Internet] 7:1048–1058. Available from: http://www.ncbi.nlm.nih.gov/pubmed/33063792
- Brzezinski D, Dauter Z, Minor W, Jaskolski M (2020) On the evolution of the quality of macromolecular models in the PDB. FEBS J. 287:2685–2698.
Pre-requisites
None.
Short bio
Prof. Wladek Minor received his Ph.D. in 1978 from the University of Warsaw in Solid State Physics. After moving to the United States in 1985 and working at Purdue University, he gradually switched to macromolecular crystallography. He joined the University of Virginia faculty in 1995. He was tenured in 1998 and promoted to full professor in 2003. In 2016, he became Harrison Distinguished Professor of Molecular Physiology and Biological Physics. He has been developing experimental protocols and computational methods for neutron scattering and X-ray diffraction since graduate school. After starting his independent career, he continued developing software within HKL, HKL-2000, and HKL-3000. He also worked on advanced solutions to other crystallographic problems: (a) Identification and refinement of metals in macromolecular structures; (b) Determination and analysis of macromolecular structures related to drug transport and drug discovery; (c) Reproducibility, ligand identification, and validation in structural biology; (d) Data mining, management, and access to primary experimental data; (e) Protocols and tools for more reliable structure determination, including the application of AI; (f) Analysis of COVID-19 structures and actions necessary to prepare for a possible future pandemic. He published more than 250 papers that attracted more than 48,000 citations. He is a co-author of more than 450 Protein Data Bank deposits. Dr. Minor has trained over 120 people that are very successful in academia, industry, and medicine. Dr. Minor’s research is often reported in general media outlets (https://minorlab.org/news), which helps taxpayers understand why investments in basic science are the best investments for the country’s future. He is elected American Association for Advancement of Science Fellow and American Crystallographic Fellow. He is also a Chair of the Commission of Biological Macromolecules of the International Union of Crystallography.