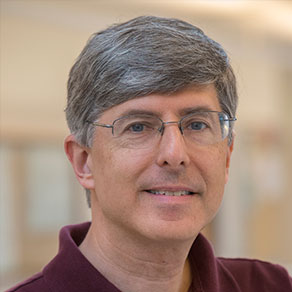
Steven Skiena
[introductory/intermediate] Word and Graph Embeddings for Machine Learning
Summary
Word/graph embedding methods (like word2vec and DeepWalk) are powerful approaches for constructing feature vectors for symbols and nodes, for use in building machine learning models in an unsupervised manner. We will review the basics of how word embeddings are constructed, graph neural networks and other approaches to building graph embeddings — along with interesting applications of these techniques to large text/network data sets.
Syllabus
- Word embeddings
- Graph embeddings
- NLP and network analysis for large datasets
References
Skiena, Steven S. The Data Science Design Manual. Springer, 2017.
Pre-requisites
None.
Short bio
Steven Skiena is Distinguished Teaching Professor of Computer Science and Director of the Institute for AI-Driven Discovery and Innovation at Stony Brook University. His research interests include data science, bioinformatics, and algorithms. He is the author of six books, including “The Algorithm Design Manual”, “The Data Science Design Manual”, and “Who’s Bigger: Where Historical Figures Really Rank”.
Skiena received his B.S. in Computer Science from the University of Virginia (Wahoo-Wa!) and his Ph.D. in Computer Science from the University of Illinois in 1988. He is the author of over 150 technical papers. He is a Fellow of the American Association for the Advancement of Science (AAAS), a current and former Fulbright scholar,
and recipient of the ONR Young Investigator Award and the IEEE Computer Science and Engineering Teaching Award. More info is available at http://www.cs.stonybrook.edu/~skiena/.