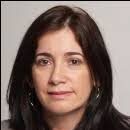
Mayte Suarez-Farinas
[intermediate] Meta-Analysis Methods for High-Dimensional Data
Summary
Meta-analysis plays an important role in summarizing and synthesizing scientific evidence derived from multiple studies. By combining multiple data sources, higher statistical power is achieved, leading to more accurate effect estimates and greater reproducibility. While the wealth of omics data in public repositories offers great opportunities to understand molecular mechanisms and identify biomarkers of human diseases, differences in design and methodology across studies often translates into poor reproducibility. Hence, the importance of meta-analytical approaches to make more robust inferences from this type of data. In this course, we will learn the most common meta-analysis strategies to integrate high-throughput biological data and to implement such analysis using R capabilities. All practical exercises will be conducted in R. Participants are encouraged to bring datasets to the course and apply the principles to their specific areas of research.
Syllabus
- Introduction to meta-analysis
- Meta-analysis of aggregated data or individual data?
- Methods to analyze aggregated data
- Sources of variation and bias specific to omics data
- Meta-analytical methods for individual omics data
References
Pre-requisites
Students must be proficient in R and familiar with the analysis of some of the omics data types. Familiarity with statistical concepts and basic understanding of regression and ANOVA. An installed version of R (https://cran.r-project.org/) and R-Studio (https://www.rstudio.com/) on a laptop is required for completing exercises.
Short bio
Mayte Suarez-Farinas, PhD, is currently a Professor at the Center for Biostatistics and the Department of Genetics and Genomics Science of the Icahn School of Medicine at Mount Sinai, New York. She received an MSc in mathematics from the University of Havana, Cuba, in year 1995, and a PhD in quantitative analysis from the Pontifical Catholic University of Rio de Janeiro, Brazil, in 2003. Prior to joining Mount Sinai, she was co-director of Biostatistics at the Center for Clinical and Translational Science at Rockefeller University, where she developed methodologies for data integration across omics studies and a framework to evaluate drug response at the molecular level in proof of concept studies in inflammatory skin diseases using mixed-effect models and machine learning. Her long term goals are to develop robust statistical techniques to mine and integrate complex high-throughput data, with an emphasis on immunological diseases, and to develop precision medicine algorithms to predict treatment response and phenotype.