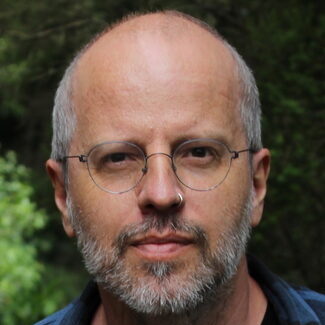
Marcelo Bertalmío
[introductory] The Standard Model of Vision and Its Limitations: Implications for Imaging, Vision Science and Artificial Neural Networks
Summary
The design of Artificial Neural Networks (ANNs) has been motivated by analogy with the brain, with neuron activity patterned after the classic single-neuron response models from vision science pioneered by Hubel and Wiesel in the 1950s and 60s, the so-called “standard model” of vision.
On the other hand, some of the well-known and most relevant problems of ANNs can be described as a failure to emulate basic human perception abilities: they need an immense amount of training data, their performance decays sharply when tested on a set different from the one used for training, and they are prone to adversarial attacks, where an image modification that is unperceptible for a human causes the ANN to completely change its response. In this regard, there is a growing number of articles pointing out the differences between ANNs and vision, and in the AI community there is a perception that deep learning as we know it might have reached its limits and that a change of paradigm is necessary, with some prominent voices advocating for using neuroscience to drive the field forward and overcome the limitations of the standard model.
In this tutorial we’ll start by going over the biological basis of vision, then we’ll discuss vision models and their limitations, and finally we’ll consider the implications for ANNs and future avenues of research.
Syllabus
- The biological basis of vision: eye and brain.
- Vision models for perception and for neural activity in the visual cortex.
- Vision research and ANNs.
References
Bertalmío, M. (2019). Vision models for high dynamic range and wide colour gamut imaging: techniques and applications. Academic Press.
Bowers, Jeffrey S., et al. (2022). Deep problems with neural network models of human vision. Behavioral and Brain Sciences: 1-74.
Bertalmío, M., Gomez-Villa, A., Martín, A., Vazquez-Corral, J., Kane, D., & Malo, J. (2020). Evidence for the intrinsically nonlinear nature of receptive fields in vision. Scientific reports, 10(1), 16277.
Saxe, A., Nelli, S., & Summerfield, C. (2021). If deep learning is the answer, what is the question? Nature Reviews Neuroscience, 22(1), 55-67.
Strickland, E. (2021). The Turbulent Past and Uncertain Future of AI: Is there a way out of AI’s boom-and-bust cycle? IEEE Spectrum, 58(10), 26-31.
Pre-requisites
Basic math knowledge.
Short bio
Marcelo Bertalmío is a Senior Research Scientist with the Spanish National Research Council (CSIC). His current research interests are in developing more accurate vision models, with applications to imaging, vision science and computer vision. He is in Stanford’s list of the world’s top scientists, ranked in the top 1% of his area. His publications total more than 14,500 citations.